Unleashing the Power of Semantic Segmentation Annotation Tool for Your Business
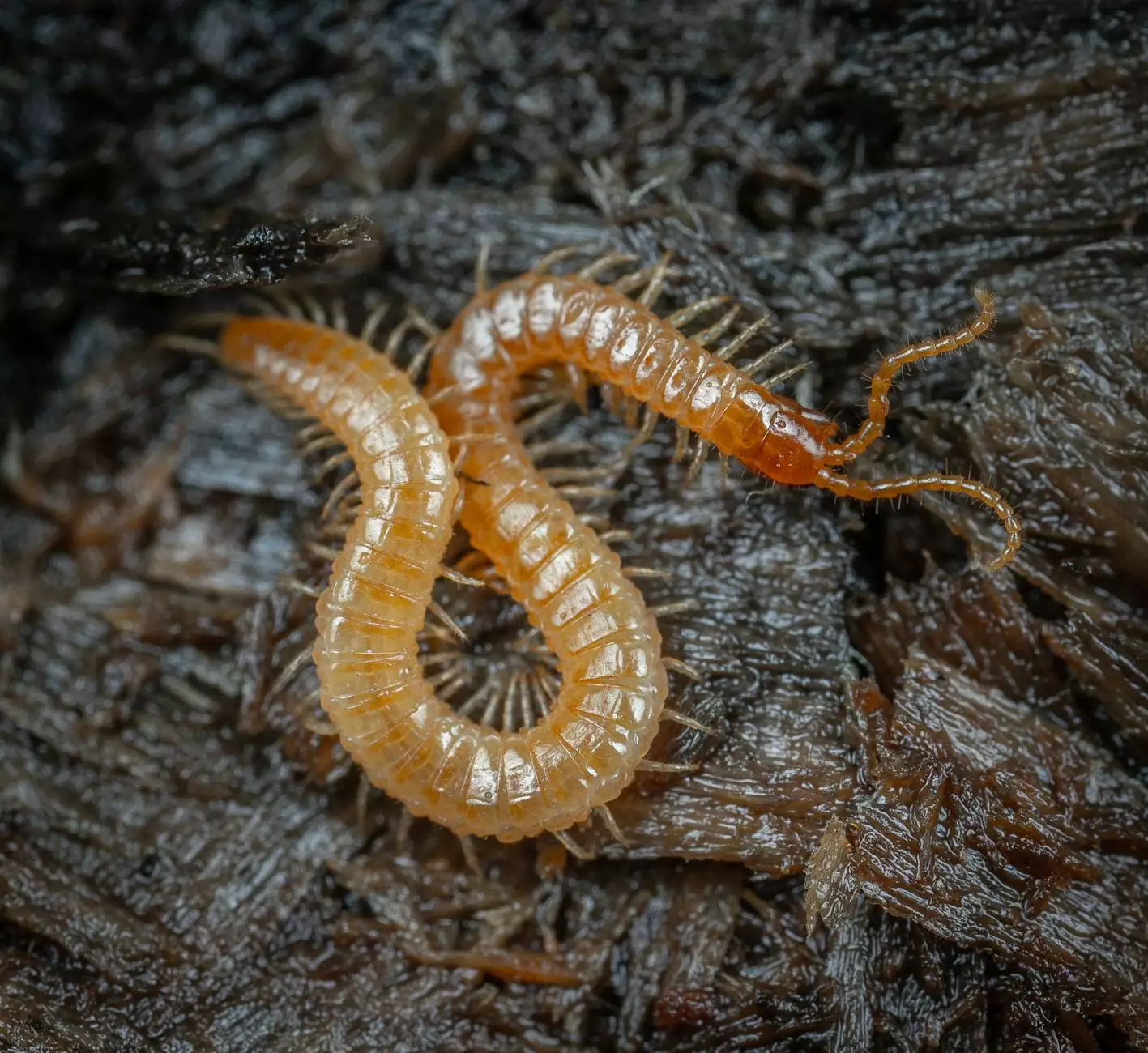
In today's fast-paced digital landscape, innovative technology is reshaping the way businesses operate and process data. One of the most significant advancements in recent times is the emergence of the semantic segmentation annotation tool. This technology is revolutionizing industries by enabling precise image analysis and improving machine learning models. In this article, we will delve deep into what semantic segmentation is, how the annotation tool works, and the profound impact it can have on your business, particularly in the realm of software development.
Understanding Semantic Segmentation
Before we explore the implications of a semantic segmentation annotation tool, it's crucial to understand what semantic segmentation actually means. At its core, semantic segmentation is a process in computer vision that involves partitioning an image into multiple segments, or pixels, and classifying each pixel into a specific category. This allows for a much more detailed understanding of the visual content.
Imagine an image containing various objects—cars, pedestrians, trees, and buildings. A semantic segmentation model will analyze this image and classify each pixel as belonging to one of these object categories. The key benefits of this approach include:
- Enhanced Accuracy: By analyzing each pixel individually, semantic segmentation provides a higher level of detail compared to traditional image classification methods.
- Contextual Understanding: This approach enables machines to understand the context of an image better, which is essential for applications like autonomous driving and robotics.
- Improved Object Interaction: Knowing the exact location of every object allows for more sophisticated interaction models, such as identifying safe zones in pedestrian-heavy images.
The Role of Semantic Segmentation Annotation Tools
The semantic segmentation annotation tool is essential for training machine learning algorithms, as it provides the labeled data needed for model training. Without proper annotations, machine learning models struggle to learn and generalize accurately. There are several advantages to using these tools:
1. Streamlined Annotation Process
Manual annotation of images can be incredibly time-consuming and prone to error. A quality semantic segmentation annotation tool automates much of this process, allowing for faster turnaround times while maintaining high accuracy. Features often include:
- Auto-Segmentation: Some tools utilize AI-driven techniques to predict and suggest initial segmentations which can be fine-tuned by human annotators.
- User-Friendly Interfaces: Many tools are designed with intuitive user interfaces that facilitate easy navigation and modification of the segmentation tasks.
- Quality Control Mechanisms: Built-in quality control methods ensure that annotations are consistent and reliable, reducing the need for extensive post-processing.
2. Diverse Use Cases Across Industries
A semantic segmentation annotation tool isn't just beneficial for one type of business; it spans various industries, including but not limited to:
- Healthcare: Used for medical image analysis, the tool can help in identifying tumors or anomalies within scans.
- Automotive: Autonomous vehicles rely heavily on high-precision mapping of the environment to operate safely.
- Retail: In e-commerce, segmenting images of products can enhance search functionality and recommendation systems.
3. Boosting Your Machine Learning Models
Data is the heart of machine learning, and semantic segmentation provides one of the richest forms of training data. Accurate segmentation allows models to:
- Learn Differentiation: Teach the model to differentiate between various object categories effectively based on pixel-wise classification.
- Achieve Higher Performance: Improved data quality often translates to better predictive accuracy and model performance.
- Enhance Transfer Learning: Pre-trained models can leverage additional annotated datasets to refine their understanding of new environments or object categories.
Choosing the Right Semantic Segmentation Annotation Tool
When selecting a semantic segmentation annotation tool, several factors must be considered to ensure it aligns with your business needs:
1. Compatibility with Your Workflow
Choose a tool that integrates seamlessly with your existing workflows. This minimizes disruption and allows your team to focus on productivity rather than adapting to new systems.
2. Customization Options
Every business has unique needs; therefore, a tool that offers customizable features for annotation styles, categories, and workflows can greatly enhance usability.
3. Scalability
Your business may grow, and so will your data needs. A good semantic segmentation annotation tool should be scalable, adapting to an increasing volume of images without compromising performance.
4. Support and Documentation
Comprehensive support and well-structured documentation can greatly assist your team in navigating the tool effectively, ensuring that you get the most out of its features.
Integrating Semantic Segmentation in Software Development
For software development companies looking to harness the power of semantic segmentation, integration can drastically enhance the capabilities of applications. Here are some key considerations:
1. Training and Fine-tuning Models
A robust understanding of how to use annotated data to train your models is essential. Deferred segmentation can lead to significant performance improvements in areas like:
- Image Classification: Enhancing the capability to classify images based on spatial attributes.
- Object Detection: Enabling machines to detect and localize multiple objects within a single image context.
2. Real-Time Applications
Many applications of semantic segmentation are real-time, such as in autonomous vehicles. Integrating the tool into your software allows your applications to make instant decisions based on visual input, proving crucial in high-stakes environments.
3. Enhancing User Experiences
By employing a semantic segmentation annotation tool effectively, businesses can create notably improved user experiences. For instance, in eCommerce, users can interact with products in a more visually appealing manner thanks to advanced image processing techniques.
Conclusion: The Future is Bright with Semantic Segmentation
The rise of the semantic segmentation annotation tool represents a significant milestone in the journey towards smarter technology. As we’ve explored, the benefits range from enhanced accuracy in image analysis to substantial time savings in data annotation processes. For companies engaged in software development, embracing this technology can lead to innovative product offerings and significantly improved machine learning models. As we step into a future increasingly dominated by AI and machine learning, the ability to annotate and understand visual data is not just an advantage—it's essential.
Choosing the right annotation tool could very well be a game changer for your business, enabling you to stay ahead of the curve and maximize your potential in the ever-competitive landscape of technology.