Revolutionizing AI with Video Annotation Tools in Computer Vision
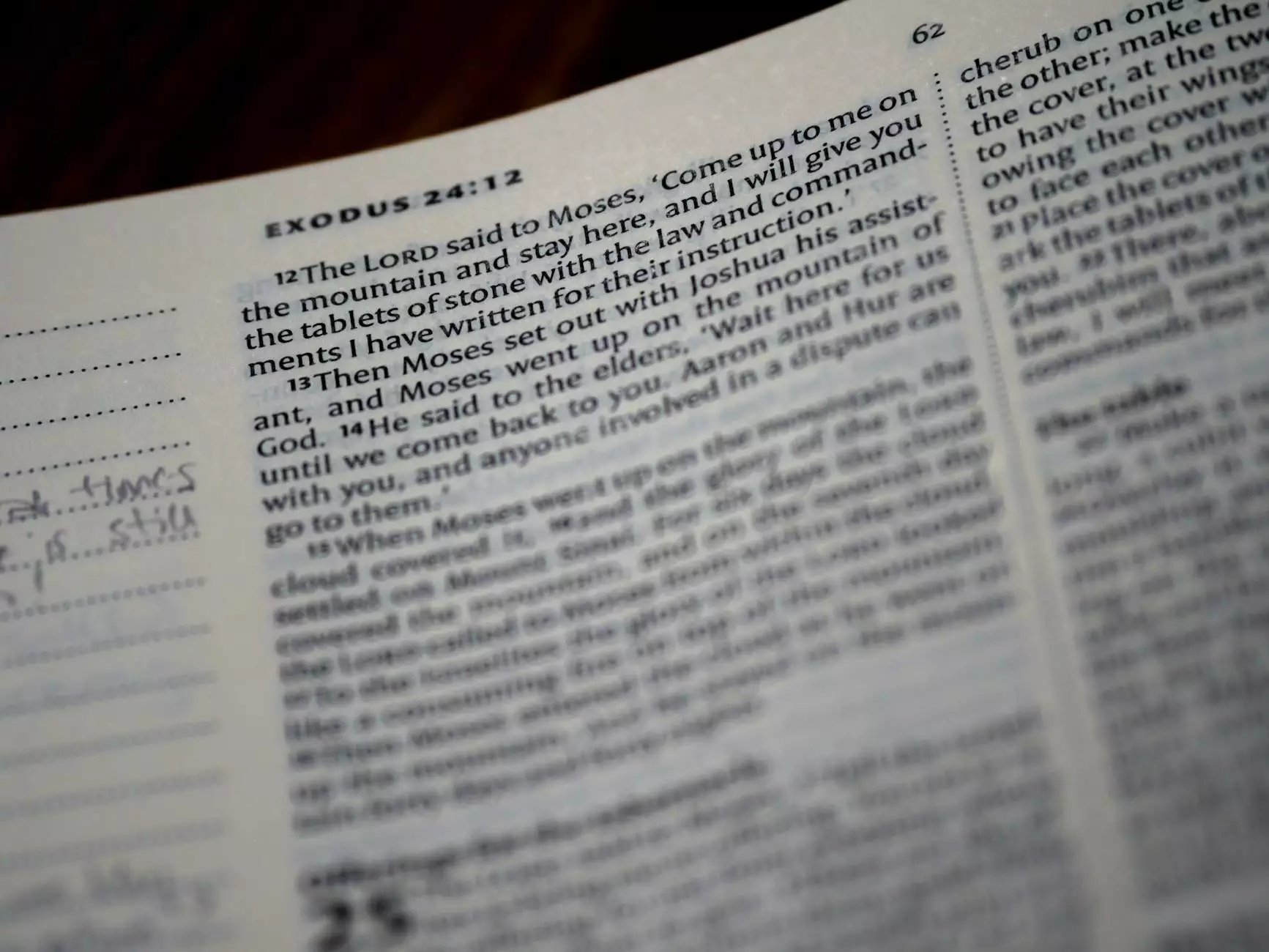
In the realm of artificial intelligence, computer vision stands out as a transformative technology enabling machines to interpret and understand the visual world. At the core of effective computer vision systems is the need for high-quality data, particularly through video annotation tools that facilitate data annotation. This article deep dives into the intricacies of video annotation, its significance, and how platforms like Keylabs.ai are leading the charge in this innovative space.
The Importance of Video Annotation in Computer Vision
Video annotation is the process of labeling video data for machine learning purposes. This crucial step allows the algorithms to learn and identify objects, actions, and events within the video footage. The significance of accurate video annotation can be summarized in the following points:
- Training AI Models: Annotated videos provide the necessary information for training robust AI models capable of real-time analysis.
- Enhancing Accuracy: High-quality annotations lead to improved predictive accuracy in various applications, from security surveillance to autonomous vehicles.
- Facilitating Advanced Research: Researchers rely on annotated data to develop and test new algorithms, pushing the boundaries of machine learning capabilities.
How Video Annotation Tools Work
Video annotation tools use various methods to label video content. Here are some common types of annotations used:
1. Bounding Boxes
Bounding boxes are rectangles drawn around objects within a video. This method is widely used in object detection tasks, where the primary goal is to locate and classify objects accurately.
2. Polygon Annotation
This method involves marking complex shapes and objects that cannot be easily enclosed within a box. Polygon annotation is beneficial for tasks requiring substantial precision, such as in autonomous driving systems.
3. Semantic Segmentation
In semantic segmentation, every pixel of the video is labeled, allowing for a more granular understanding of the scene. This technique is particularly useful in applications like medical imaging and urban planning.
4. Action Recognition
Action recognition annotations label specific actions occurring in the video, making them vital for applications in sports analytics and human-computer interaction.
Benefits of Using a Video Annotation Tool
Utilizing a video annotation tool offers numerous advantages that can significantly enhance the quality and efficiency of the data annotation process:
- Scalability: Video annotation tools can handle vast amounts of data, allowing businesses to scale their AI projects seamlessly.
- Collaboration: Many annotation platforms promote teamwork by enabling multiple annotators to work on projects simultaneously, ensuring faster turnaround times.
- Quality Control: Advanced tools often come with built-in quality control mechanisms, allowing for the review and improvement of annotations, leading to higher data integrity.
Key Features of Leading Annotation Platforms
When selecting a video annotation tool, several critical features should be considered:
1. User-friendly Interface
A simple and intuitive interface can drastically reduce the learning curve for new users, making it easier for teams to adopt the tool quickly.
2. Customizable Annotation Tools
Flexibility to add custom annotation types ensures that specific project requirements can be met effectively, whether it’s simple bounding boxes or complex polygon annotations.
3. Integration Capabilities
Seamless integration with existing systems and workflows is crucial for maintaining productivity. A good video annotation tool should support various APIs and plugins.
4. Advanced Analytics
Advanced reporting features can help teams understand annotation progress and identify areas for improvement, ensuring a streamlined workflow.
Keylabs.ai: A Leader in Video Annotation Tool for Computer Vision
Keylabs.ai stands out as a comprehensive solution for businesses looking to harness the power of video annotation in computer vision. Here’s how Keylabs.ai excels:
1. Cutting-Edge Technology
Incorporating the latest advancements in AI and machine learning, Keylabs.ai offers robust annotation tools capable of enhancing the speed and accuracy of the data preparation process.
2. Versatile Applications
From autonomous vehicles and security systems to healthcare and retail, Keylabs.ai provides customized solutions tailored to various industries requiring precise video annotation.
3. Expert Support
Keylabs.ai prides itself on offering exceptional support to its users, with a team of experts always ready to assist and guide clients through the annotation process.
Case Studies: Success Stories with Video Annotation
Numerous organizations have successfully implemented video annotation tools to enhance their operations. Here are a few noteworthy examples:
1. Autonomous Vehicle Development
A leading automotive manufacturer used video annotation tools to label driving footage, resulting in enhanced object detection algorithms. This improved the safety and efficiency of their self-driving cars significantly.
2. Security and Surveillance
A security company utilized video annotation to analyze footages and identify suspicious activities more accurately, which led to a substantial reduction in crime rates in monitored areas.
3. Medical Imaging
In healthcare, a research group employed video annotation to assist in diagnosing medical conditions from patient interactions and movements, streamlining their patient care processes.
Challenges in Video Annotation and How to Overcome Them
Despite the advantages, organizations may face challenges while implementing video annotation tools. Here are some common issues and proposed solutions:
1. High Annotation Costs
Video annotation can be time-consuming and expensive. To mitigate this, companies can leverage automated annotation tools available in platforms like Keylabs.ai, which reduce manual effort significantly.
2. Ensuring Annotation Quality
Quality can vary among annotators, leading to inconsistencies. Implementing rigorous quality control processes, including regular checks and validation tests, is essential for maintaining high standards.
3. Handling Large Datasets
Large volumes of video data can overwhelm teams. Adopting scalable solutions and integrating machine learning for initial annotations can help streamline the process and maintain efficiency.
Future Trends in Video Annotation for Computer Vision
As technology evolves, the future of video annotation will continue to be dynamic, with several trends emerging:
- Increased Automation: Abuse of AI will automate many aspects of video annotation, drastically reducing the time required for initial labeling.
- Real-time Annotation: As computer vision technology advances, we will see more tools capable of real-time video annotation, allowing for instant data processing and analysis.
- Collaboration with Influencers: Collaborations between AI companies and influencers in various fields can lead to more enriched data sets through shared insights and feedback loops.
Conclusion
In conclusion, the integration of video annotation tools in computer vision is not merely an enhancement; it is a necessity in the rapidly evolving world of artificial intelligence. With platforms like Keylabs.ai, businesses can ensure that their data annotation processes are not only efficient but also produce high-quality datasets that drive successful AI models. The continuous improvement in technology, along with a keen focus on user experience, will undoubtedly make video annotation tools integral to the future of computer vision and AI development.
video annotation tool computer vision