The Evolution of Image Annotation Tools: A Comprehensive Guide
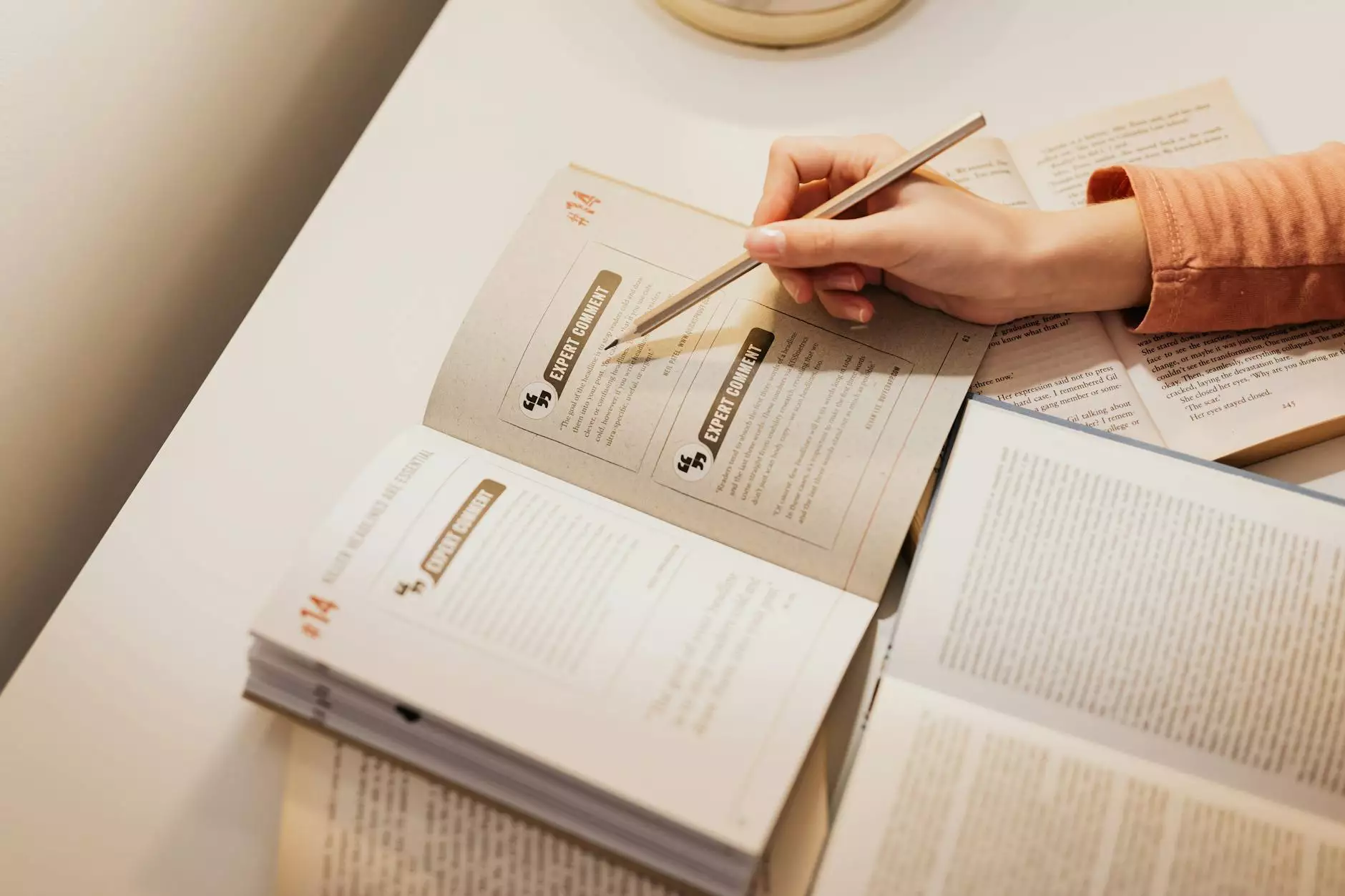
In the contemporary landscape of data-driven technology, the significance of image annotation tools cannot be overstated. These tools serve as the backbone of machine learning and artificial intelligence, enabling algorithms to understand and interpret visual data accurately. At KeyLabs.ai, we deeply understand the nuances of image annotation tools and how they can transform the way businesses interact with their data.
What is Image Annotation?
Image annotation refers to the process of labeling images so that a machine learning model can learn from them. This practice is essential for training various algorithms in tasks such as object detection, image classification, and segmentation. By providing context to the visual data, businesses can enhance the accuracy of their machine learning models.
Why are Image Annotation Tools Crucial?
In a world where image data is exploding in volume, the necessity for efficient annotation processes is greater than ever. Here are some reasons why image annotation tools are critical:
- Enhancing Data Quality: High-quality annotated data leads to better-performing models.
- Speeding Up the Annotation Process: Automated tools can save significant time compared to manual annotation.
- Scalability: Businesses can handle large datasets effortlessly with the right tools.
- Consistency: Tools ensure uniformity in the annotation process, minimizing human error.
Types of Image Annotation Tools
Various types of image annotation tools cater to different needs. Here are some common options:
1. Bounding Box Annotation Tools
Bounding box annotation tools allow users to create rectangular boxes around objects within an image, making them particularly effective for object detection tasks. Examples include:
- KeyLabs.ai Annotation Tool: Ideal for various bounding box applications.
- VGG Image Annotator: An open-source tool widely used in research.
2. Polygon Annotation Tools
For more complex shapes, polygon annotation tools are used. These tools allow for outlining non-rectangular objects, enhancing accuracy in segmentation tasks. Some popular options include:
- LabelMe: A community-driven tool for polygonal annotations.
- MakeSense.ai: A user-friendly interface for polygon annotations.
3. Semantic Segmentation Tools
Semantic segmentation tools label each pixel of an image, allowing for finer granularity in data representation. Tools in this category include:
- Supervisely: Offers advanced capabilities for semantic segmentation.
- Labelbox: Provides an intuitive platform for managing segmentation projects.
The Benefits of Using Image Annotation Tools
Choosing the right image annotation tools can significantly enhance productivity and the quality of your datasets:
1. Increased Efficiency
By automating the annotation process, businesses can drastically reduce the time taken to prepare datasets for training machine learning models. This automation allows teams to focus on analysis and model refinement rather than the tedious task of manual labeling.
2. Better Accuracy
Tools often come with built-in quality control measures, ensuring that the annotations are not only fast but also precise. This level of accuracy is especially important in critical applications such as autonomous vehicles or medical imaging.
Choosing the Right Image Annotation Tool
When selecting an image annotation tool, it’s crucial to consider various factors to ensure it meets your business needs:
- User-Friendly Interface: The tool should be easy to navigate for all team members.
- Integration Capabilities: Ensure it can seamlessly integrate with your existing workflows and systems.
- Scalability: Choose a tool that can scale with your data needs as your business grows.
- Cost-Effectiveness: Analyze pricing models and select one that fits your budget while offering the necessary features.
Best Practices for Image Annotation
To maximize the effectiveness of your image annotation efforts, consider adopting these best practices:
1. Define Clear Guidelines
Establish clear guidelines for annotators to ensure consistency. This includes defining what constitutes different labels, the level of detail required, and how ambiguity should be handled.
2. Utilize Quality Control Measures
Implement regular quality checks to identify any discrepancies in the annotations. Tools with built-in review processes can facilitate this.
3. Provide Comprehensive Training
Invest time in training your annotators. Familiarity with the tools and understanding of the project objectives significantly improves quality and efficiency.
The Future of Image Annotation Tools
The field of image annotation is continuously evolving. With innovations in AI and machine learning, we can expect:
- Automated Annotation: Advanced algorithms may soon automate much of the annotation process, reducing the need for manual input.
- Real-Time Collaboration: Cloud-based tools will allow for streamlined real-time collaboration among teams across the globe.
- Enhanced AI Integration: Improved AI might assist annotators by suggesting labels or detecting potential errors.
Conclusion
In conclusion, leveraging the right image annotation tools is essential for any business aiming to harness the power of machine learning and artificial intelligence. Tools like those offered at KeyLabs.ai enable companies to annotate data efficiently, enabling the development of accurate and reliable predictive models. By investing in these tools and adhering to best practices, businesses can ensure they stay ahead in the competitive landscape of technology. Embrace the future of data annotation today, and unlock the full potential of your visual data.